Generative AI and Its Economic Impact: What You Need to Know
Our estimates intuitively suggest that fewer jobs in EMs are exposed to automation than in DMs, but that 18% of work globally could be automated by AI on an employment-weighted basis. … Our scenario analysis suggests that the ultimate share of work exposed to automation could range from 15-35%, a range which is consistent with—but on the conservative side of—existing estimates in the literature. Generative AI may have just recently hit the mainstream, but the economics of GenAI already point to a shift in the industry’s balance of power, away from the dominant tech giants.
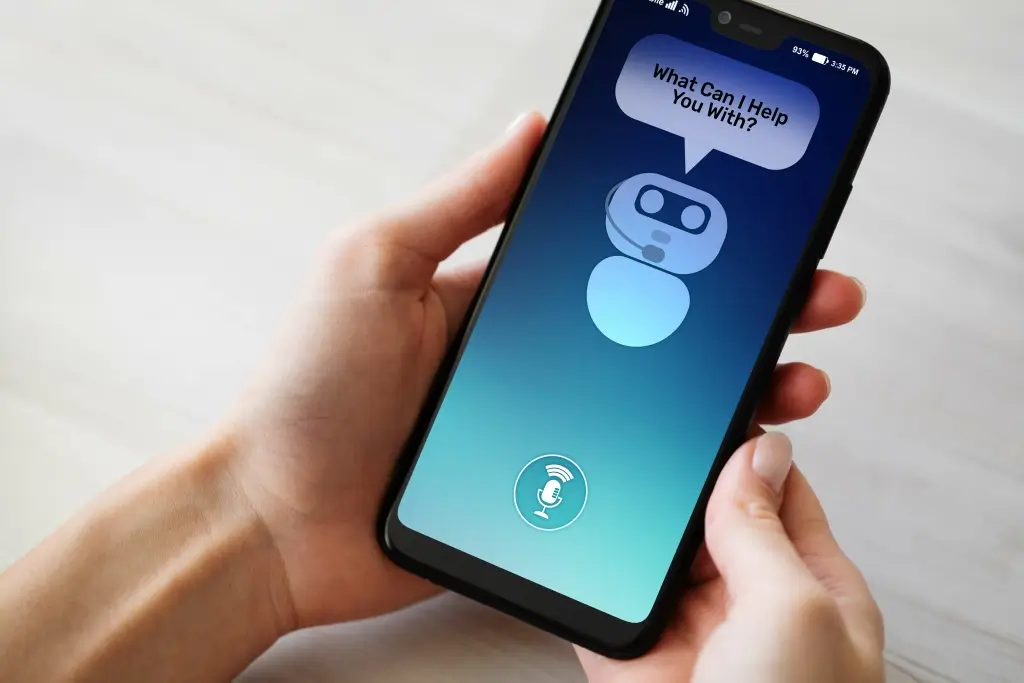
In the near future, we expect applications that target specific industries and functions will provide more value than those that are more general. Generative AI has the potential to automate certain tasks, displacing some workers, and it can also create new jobs and industries. The exact impact of AI on jobs is difficult to predict and will likely vary depending on the industry and the specific tasks involved. A study by Accenture found that artificial intelligence could add $14 trillion to the global economy by 2035, with the most significant gains in China and North America.
Apps keep proliferating to address specific use cases
Our second lens complements the first by analyzing generative AI’s potential impact on the work activities required in some 850 occupations. We modeled scenarios to estimate when generative AI could perform each of more than 2,100 “detailed work activities”—such as “communicating with others about operational plans or activities”—that make up those occupations across the world economy. This enables us to estimate how the current capabilities of generative AI could affect labor productivity across all work currently done by the global workforce. Some of this impact will overlap with cost reductions in the use case analysis described above, which we assume are the result of improved labor productivity.
Still, our research indicates the technology could deliver productivity with a value ranging from 10 to 15 percent of overall R&D costs. For one thing, mathematical models trained on publicly available data without sufficient safeguards against plagiarism, copyright violations, and branding recognition risks infringing on intellectual property rights. A virtual try-on application may produce biased representations of certain demographics because of limited or biased training data. Thus, significant human oversight is required for conceptual and strategic thinking specific to each company’s needs. It does not account for potential knock-on effects the technology may have on customer satisfaction and retention arising from an improved experience, including better understanding of the customer’s context that can assist human agents in providing more personalized help and recommendations. The latest generative AI applications can perform a range of routine tasks, such as the reorganization and classification of data.
Global economist to speak about economic potential of Generative AI
Generative AI has taken hold rapidly in marketing and sales functions, in which text-based communications and personalization at scale are driving forces. The technology can create personalized messages tailored to individual customer interests, preferences, and behaviors, as well as do tasks such as producing first drafts of brand advertising, headlines, slogans, social media posts, and product descriptions. We analyzed only use cases for which generative AI could deliver a significant improvement in the outputs that drive key value.
Its ability to rapidly digest mountains of data and draw conclusions from it enables the technology to offer insights and options that can dramatically enhance knowledge work. This can significantly speed up the process of developing a product and allow employees to devote more time to higher-impact tasks. Following are four examples of how generative AI could produce operational benefits in a handful of use cases across the business functions that could deliver most of the potential value we identified in our analysis of 63 generative AI use cases.
Pharma companies typically spend approximately 20 percent of revenues on R&D,1Research and development in the pharmaceutical industry, Congressional Budget Office, April 2021. With this level of spending and timeline, improving the speed and quality of R&D can generate substantial value. For example, lead identification—a step in the drug discovery process in which researchers identify a molecule that would best address the target for a potential new drug—can take several months even with “traditional” deep learning techniques. Foundation models and generative AI can enable organizations to complete this step in a matter of weeks.
Very roughly speaking, for each additional word of response from an LLM, the entire cumulative conversation needs to be passed through the entire model. The cost of this inference—which is how an LLM or LMM generates its output—is therefore proportional to the number of parameters in the model multiplied by the number of words of the conversation. Accountability has to be a core principle, to ensure that machines remain subject to effective oversight by people. Therefore, as generative AI capability grows, more organizations the economic potential of generative ai will need workers who oversee the reliable, fair, and ethical use of the technology. “There will still need to be human judgment to account for potential algorithmic bias, as well as person-to-person interaction to manage important stakeholder relationships,” Mazhari explains. For example, in India, where the number of individual creators is already on the rise, a survey of more than 1,600 freelancers found that 47% were using generative AI tools regularly and more than 50% reported a positive impact on their productivity.
Adoption is also likely to be faster in developed countries, where wages are higher and thus the economic feasibility of adopting automation occurs earlier. Even if the potential for technology to automate a particular work activity is high, the costs required to do so have to be compared with the cost of human wages. In countries such as China, India, and Mexico, where wage rates are lower, automation adoption is modeled to arrive more slowly than in higher-wage countries (Exhibit 9). In the lead identification stage of drug development, scientists can use foundation models to automate the preliminary screening of chemicals in the search for those that will produce specific effects on drug targets.
Turbo-charging productivity in Asia: the economic benefits of generative AI – MIT Technology Review
Turbo-charging productivity in Asia: the economic benefits of generative AI.
Posted: Wed, 05 Jul 2023 07:00:00 GMT [source]
If the past eight months are any guide, the next several years will take us on a roller-coaster ride featuring fast-paced innovation and technological breakthroughs that force us to recalibrate our understanding of AI’s impact on our work and our lives. Given the speed of generative AI’s deployment so far, the need to accelerate digital transformation and reskill labor forces is great. These tools have the potential to create enormous value for the global economy at a time when it is pondering the huge costs of adapting and mitigating climate change.
Our estimates are based on the structure of the global economy in 2022 and do not consider the value generative AI could create if it produced entirely new product or service categories. Four months later, OpenAI released a new large language model, or LLM, called GPT-4 with markedly improved capabilities.1“Introducing ChatGPT,” OpenAI, November 30, 2022; “GPT-4 is OpenAI’s most advanced system, producing safer and more useful responses,” OpenAI, accessed June 1, 2023. My role in this evolution has been as much about learning and adapting as it has been about leading a company in this field.
McKinsey’s report is one of the few so far to quantify the long-term impact of generative A.I. Tools like ChatGPT and Google’s Bard, with tech companies and venture capitalists investing billions of dollars in the technology. Based on a historical analysis of various technologies, we modeled a range of adoption timelines from eight to 27 years between the beginning of adoption and its plateau, using sigmoidal curves (S-curves).
Companies, policymakers, consumers, and citizens can work together to ensure that generative AI delivers on its promise to create significant value while limiting its potential to upset lives and livelihoods. Combining generative AI with all other technologies, work automation could add 0.5 to 3.4 percentage points annually to productivity growth. In 2012, the McKinsey Global Institute (MGI) estimated that knowledge workers spent about a fifth of their time, or one day each work week, searching for and gathering information. If generative AI could take on such tasks, increasing the efficiency and effectiveness of the workers doing them, the benefits would be huge. Such virtual expertise could rapidly “read” vast libraries of corporate information stored in natural language and quickly scan source material in dialogue with a human who helps fine-tune and tailor its research, a more scalable solution than hiring a team of human experts for the task. In other cases, generative AI can drive value by working in partnership with workers, augmenting their work in ways that accelerate their productivity.
We also modeled a range of potential scenarios for the pace at which these technologies could be adopted and affect work activities throughout the global economy. A third major area of economic impact involves enhancing workplace efficiency through generative AI’s ability to digest and summarize vast amount of information. The technology helps to make big data more interpretable and useful for decision-making, especially in industries that rely on large amounts of data or involve complex tasks, such as financial services, professional services, scientific research, and ICT. But equally, generative AI tools offer productivity benefits for workers in administrative fields—lessening their workloads and enabling them to refocus on higher-level or more interpersonally challenging work. Gen AI’s precise impact will depend on a variety of factors, such as the mix and importance of different business functions, as well as the scale of an industry’s revenue.
- Once promoted, everybody thinks their chance of getting the next promotion goes down because of their race.
- Our analysis of 16 business functions identified just four—customer operations, marketing and sales, software engineering, and research and development—that could account for approximately 75 percent of the total annual value from generative AI use cases.
- Thus, significant human oversight is required for conceptual and strategic thinking specific to each company’s needs.
- “Skilling programs exist today in pockets across Asia, but too many people are severely underserved because of race, gender, geography, displacement, or other barriers,” Mazhari says.
My journey with AI, from its academic origins to becoming a business necessity, reflects the larger trend of AI’s evolution. It’s a field that continues to grow and surprise, and I’m excited to see where the next breakthrough will lead us. Inspired by the classic “enhance” command in crime shows, this ability resonated with the public’s imagination in terms of AI.
On top of that impact, the use of generative AI tools could also enhance customer satisfaction, improve decision making and employee experience, and decrease risks through better monitoring of fraud and risk. Generative AI tools can enhance the process of developing new versions of products by digitally creating new designs rapidly. A designer can generate packaging designs from scratch or generate variations on an existing design. In the banking industry, generative AI has the potential to improve on efficiencies already delivered by artificial intelligence by taking on lower-value tasks in risk management, such as required reporting, monitoring regulatory developments, and collecting data. In the life sciences industry, generative AI is poised to make significant contributions to drug discovery and development. Our analysis did not account for the increase in application quality and the resulting boost in productivity that generative AI could bring by improving code or enhancing IT architecture—which can improve productivity across the IT value chain.
- The expected business disruption from gen AI is significant, and respondents predict meaningful changes to their workforces.
- To address those risks, many countries—such as the United States, Australia, and China—have launched initiatives to create frameworks of regulations and policies for AI, and some have expanded their existing AI regulations to explicitly include gen AI, too.
- Some 40 percent of the activities that workers perform in the economy require at least a median level of human understanding of natural language.
- A virtual try-on application may produce biased representations of certain demographics because of limited or biased training data.
- But a full realization of the technology’s benefits will take time, and leaders in business and society still have considerable challenges to address.